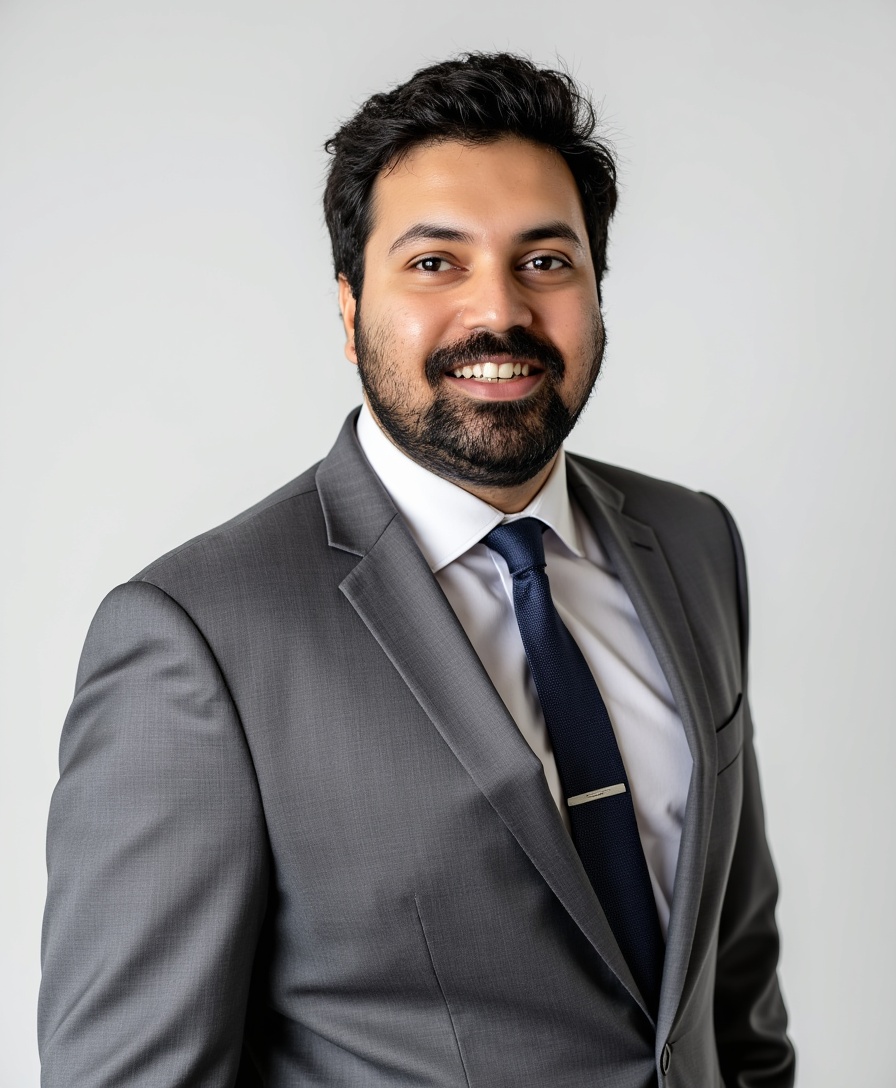
Resume: Sahidul Islam
Assistant Professor
Email: sislam19[@]kennesaw[.]edu
Interest
Music, Nature, ExploreAffiliation
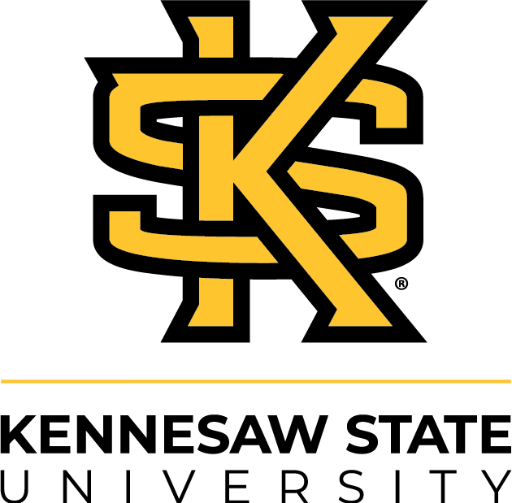
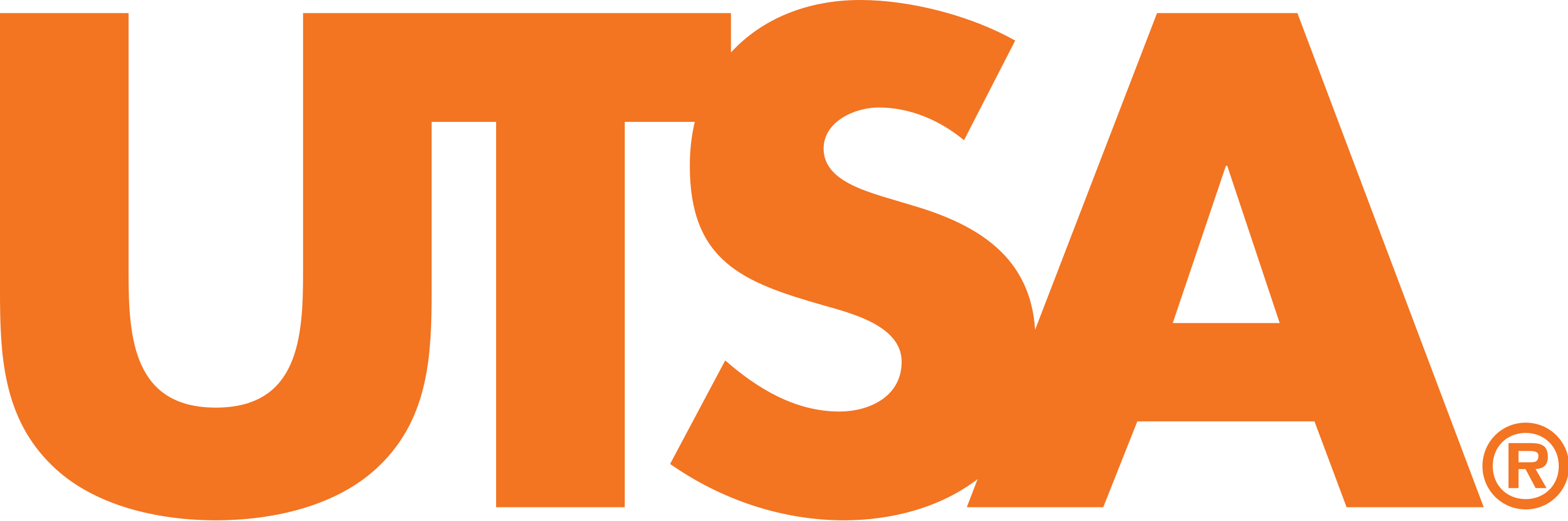
Research Projects (under construction ....)
On-device Life Long learning
How can we address catastrophic forgetting and evaluate learning, especially when labeled data is unavailable?
In real-world applications, lifelong learning offers the potential for systems to adapt continuously, make informed decisions over time, and improve without requiring complete retraining from scratch.
In real-world applications, lifelong learning offers the potential for systems to adapt continuously, make informed decisions over time, and improve without requiring complete retraining from scratch.
Secure MPC based Privacy Preserving Machine Learning
Can we solve non plynomial convincingly in MPC?
Secure Multi-Party Computation (MPC) enables privacy-preserving machine learning by allowing multiple parties to collaboratively train models or perform inference on encrypted data without revealing their individual inputs. While the mathematical foundations of MPC guarantee privacy and accuracy for polynomial functions, non-polynomial components, such as the rectified linear unit (ReLU) activation function, remain challenging. Although ReLU is widely used in the plaintext domain of DNNs, in the ciphertext domain, it requires multiple rounds of communication, encoding, and decoding between parties due to its reliance on the oblivious transfer (OT) protocol.
Secure Multi-Party Computation (MPC) enables privacy-preserving machine learning by allowing multiple parties to collaboratively train models or perform inference on encrypted data without revealing their individual inputs. While the mathematical foundations of MPC guarantee privacy and accuracy for polynomial functions, non-polynomial components, such as the rectified linear unit (ReLU) activation function, remain challenging. Although ReLU is widely used in the plaintext domain of DNNs, in the ciphertext domain, it requires multiple rounds of communication, encoding, and decoding between parties due to its reliance on the oblivious transfer (OT) protocol.
DNN Inference on Resource Constraint System
Deep Neural Network (DNN) inference on edge devices allows for real-time processing and decision-making directly on the device, without relying on cloud connectivity.
This approach enhances privacy, reduces latency, and conserves bandwidth by keeping data and computations local.
Despite the resource constraints of edge devices, optimizations like model compression and quantization make DNN inference feasible and efficient.
Applied Machine learning
Applied machine learning involves using machine learning techniques to solve real-world problems by developing models that can learn from data and make predictions or decisions.
It spans a wide range of applications, from healthcare to transportation.
The focus is on translating theoretical concepts into practical solutions that deliver tangible benefits in various industries.